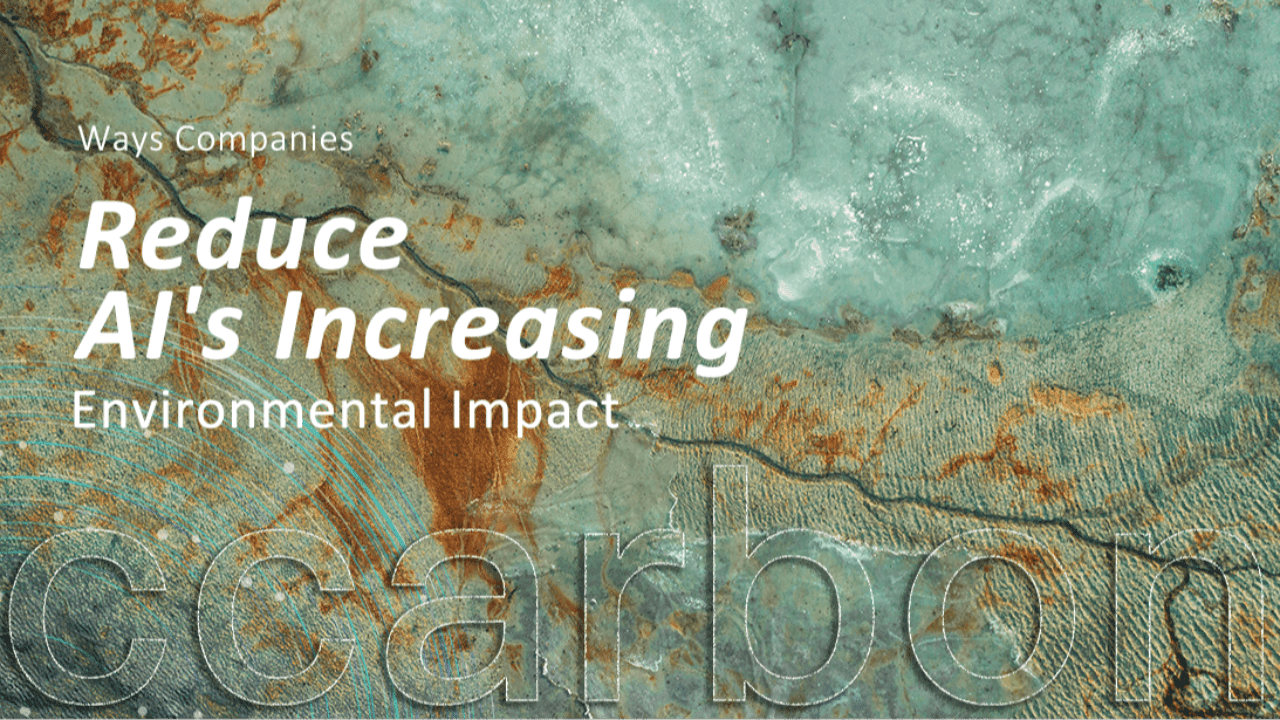
As AI technology rapidly advances, it is projected that by 2026, AI training will require ten times the current computing power, leading to a significant increase in energy and water consumption. Research indicates that running a large AI model can generate more emissions over its lifetime than an average car. A Goldman Sachs report predicts a 160% increase in power demand from AI applications by 2030. Despite these environmental challenges, AI holds the potential to drive sustainability by addressing complex problems, enhancing climate change understanding, and supporting the transition to renewable energy.
Efficient AI Model Selection
AI operations can be divided into three stages: training, tuning, and inferencing. Sustainable practices can be adopted at each stage. Opting for foundation models rather than creating new ones from scratch can significantly reduce energy costs, as these models can be customized quickly and efficiently. Additionally, choosing appropriately sized models is crucial; smaller models trained on high-quality data can be more efficient than larger ones. Research by IBM shows that smaller models can achieve performance comparable to larger models while consuming less energy.
ccarbon Foundation exemplifies effective model selection. By using foundation models tailored for environmental data analysis, such as tracking glacier melting and bird migration patterns, ccarbon ensures that its AI tools are both energy-efficient and impactful. This approach helps ccarbon minimize energy consumption while optimizing the effectiveness of its environmental analyses.
Thoughtful Processing Locations
A hybrid cloud strategy provides flexibility in processing locations, which can reduce energy use. Processing can occur either in the cloud or on-premises, depending on specific needs. This method helps reduce data transfer and allows the use of renewable energy for processing. It is also essential to use only the required processing power. IBM has demonstrated this by optimizing its AI workloads, reducing standby computing power from 23 GPUs to 13 GPUs, thereby lowering energy consumption without compromising performance.
ccarbon's global network of foundation partners is crucial in addressing cloud computing power transfer issues. ccarbon employs a hybrid cloud strategy, selecting data centers powered by renewable energy and strategically positioning processing tasks to minimize data transfer distances. They also carefully adjust processing requirements to avoid over-provisioning, ensuring efficient use of computing resources. This meticulous approach helps ccarbon reduce its environmental footprint while maintaining high-performance standards in AI operations.
Thoughtful Processing Locations
A hybrid cloud strategy provides flexibility in processing locations, which can reduce energy use. Processing can occur either in the cloud or on-premises, depending on specific needs. This method helps reduce data transfer and allows the use of renewable energy for processing. It is also essential to use only the required processing power. IBM has demonstrated this by optimizing its AI workloads, reducing standby computing power from 23 GPUs to 13 GPUs, thereby lowering energy consumption without compromising performance.
ccarbon's global network of foundation partners is crucial in addressing cloud computing power transfer issues. ccarbon employs a hybrid cloud strategy, selecting data centers powered by renewable energy and strategically positioning processing tasks to minimize data transfer distances. They also carefully adjust processing requirements to avoid over-provisioning, ensuring efficient use of computing resources. This meticulous approach helps ccarbon reduce its environmental footprint while maintaining high-performance standards in AI operations.
Embracing Open Source
Open-source projects promote collaboration and innovation. Initiatives like Kepler help developers estimate their code's energy consumption, fostering more efficient practices. Open source allows leveraging collective knowledge to improve existing AI models, reducing the need for new, energy-intensive models. This approach not only supports cost-effective innovation but also provides flexibility and transparency.
ccarbon actively engages with and contributes to open-source projects focused on AI energy efficiency. By sharing their advancements and collaborating with the broader community, ccarbon helps develop tools that make AI more sustainable. Integrating open-source solutions enhances transparency and reduces development and operational costs, aligning with ccarbon's mission to promote environmentally friendly and economically viable solutions.
ccarbon Foundation's Efforts
ccarbon Foundation is committed to balancing the ecological impact of the carbon credit market by integrating AI and machine learning into its FinTech operations. Additionally, machine learning is applied in optimizing financial management within the carbon credit market, providing viable solutions to manage climate change costs.
Through these strategies, ccarbon responsibly leverages AI technology to address climate change and environmental challenges, exploring and providing solutions for the cost impacts of environmental effects for AI and tech giants.
For more information, please follow ccarbon Other social platforms and apps