The ability to simulate the world (World Model) is regarded by some experts as the next major step for AI to be able to 'perceive' and recreate the physical world.
Some companies or laboratories are pushing the capability of world modeling for AI. Among them, Professor Fei-Fei Li's World Labs, one of the pioneers in AI, raised $230 million to build a 'large world model'. Google DeepMind has also hired Tim Brooks, the head of the Sora team, and another expert, William Peebles, to develop a 'world simulator'.
"The images of the world around us that we hold in our heads are merely models. No one in their head can imagine the entire world, government, or country. People only select concepts and the relationships between them and use them to represent the actual system," according to the definition of Mental Model as stated in the book 'The Counterintuitive Behavior of Social Systems' written by Jay Wright Forrester, an American computer engineer, management theorist, and systems scientist in 1971.
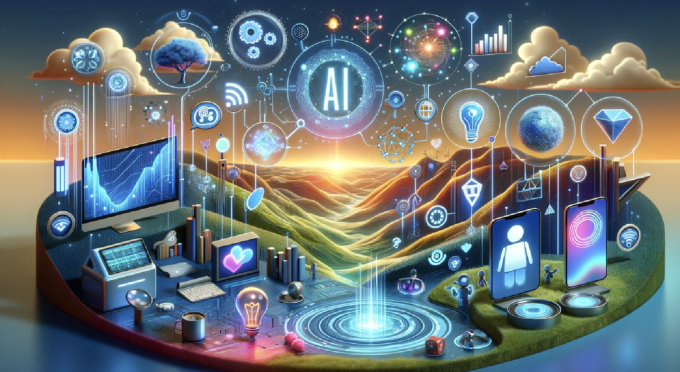
Illustration of the 'world of AI'. Photo: Novita
World Models are seen as an evolution from Mental Models, both inspired by the human brain. The brain takes abstract representations from the senses, forming a specific understanding of the surrounding world. The predictions made by the brain are based on models that influence how a person perceives the world.
Two researchers, David Ha and Jürgen Schmidhuber, cited the example of how baseball players play. These individuals have only a thousandth of a second to decide how to swing the bat, and this timeframe is shorter than the time needed to send signals from vision to the brain. To do this, they need to predict how the ball will be thrown and its trajectory before it arrives.
"This is the aspect for AI to reach human-level capability if applying World Models," Ha and Schmidhuber wrote in a joint report posted on Github.
According to experts, AI that generates video from text, like Sora, falls into what is called the 'uncanny valley'. This means that videos generated by AI still contain many errors, especially with fast motion, partly because the algorithms cannot predict the next 'model' as the human brain does.
According to TechCrunch, current AI video generation tools can accurately predict a bouncing basketball, but in reality, they do not know the reason why. Similarly, language models do not truly understand the concepts behind words and phrases.
However, World Models make AI truly intelligent by 'understanding' why a ball bounces. To gain that deep understanding, World Models need to be trained on various types of data such as images, sounds, videos, and texts, with the aim of creating internal reasoning about how the world operates and the ability to interpret the outcomes of actions.
"Viewers expect what they see to behave like it does in reality," Alex Mashrabov, former AI director of Snap and CEO of the world modeling company Higgsfield, told TechCrunch. "A sufficiently powerful World Model will understand how objects move instead of waiting for the creator to 'draw the path' for it to move."
But creating better videos is just one aspect of the application of World Models. Leading AI researchers, like Yann LeCun, Meta's AI director, predict that one day, they could be used for forecasting and sophisticated planning in both digital and physical domains.
At the beginning of this year, LeCun described how World Models can help an AI system achieve desired goals through reasoning. A model presents an initial scenario, for example, a video of a dirty room, gives it the goal of a clean room, and a series of actions to achieve that goal, such as deploying a vacuum cleaner to sweep, washing dishes, and taking out the trash. In this process, the AI not only recognizes through cameras and sensors but also 'knows' at a deeper level how to transition from dirty to clean.
"We need machines that understand the world, can remember everything, have intuition, have common sense - things that can reason and plan at a human level," LeCun said. "Current AI systems cannot do any of that. It may take another decade for them to emerge."
OpenAI stated that Sora can be considered a primitive World Model as it simulates actions, such as an artist leaving brush strokes on a canvas. However, the company also acknowledges that it will take a long time to perfect the feature.
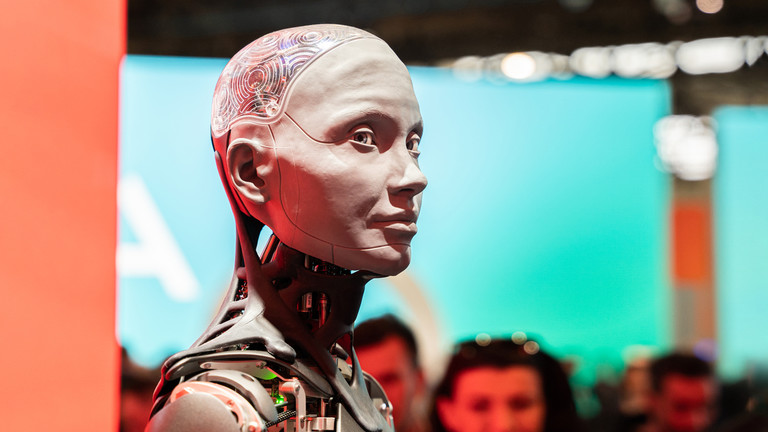
Despite the great potential, building World Models is costly, as it requires enormous computational power compared to current capabilities. It is estimated that even a small tool could consume thousands of the most powerful GPUs for training.
Moreover, the input data for World Models is also many times larger than that of existing large language models. "The training data for the model must be broad enough to cover diverse scenarios but very specific for AI to deeply understand the nuances of those scenarios," commented Mashrabov from Higgsfield. "The lack of data is slowing down advances."
Cristóbal Valenzuela, CEO of Runway AI, also believes data is the biggest barrier on the path to building World Models. "Models need a lot of data and techniques to create a consistent map of the environment, the ability to navigate and interact within that environment," Valenzuela wrote on his blog.
However, Mashrabov believes that if these barriers are overcome, World Models will be 'more powerful' in connecting AI with the real world, especially when combined with robotics.
"Today's robots are limited in their ability to perform tasks because they do not perceive their surroundings. World Models can provide them with that capability," he said. "With an advanced model, AI can develop personal understanding of any scenario it is placed in and begin to reason feasible solutions."