introduction
The rapid development of artificial intelligence (AI) has brought revolutionary changes in many fields, but problems have also gradually emerged. One of the key issues is the "hallucination" phenomenon of AI models, that is, the tendency of models to produce high-confidence outputs without training data. This problem is particularly significant in large language model (LLM) research. However, a team of scientists from the University of Science and Technology of China (USTC) and Tencent Youtu Lab recently developed a tool called "Woodpecker" to correct this problem. This article will introduce in detail the technology behind the Woodpecker tool, its application, and its significant improvements in transparency and accuracy of AI models.
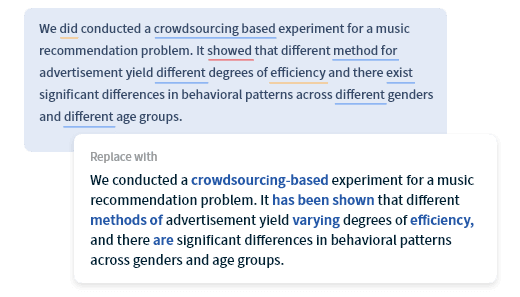
The “hallucination” phenomenon of AI models
Before discussing the Woodpecker tool, let’s first understand the phenomenon of “hallucination” in AI models. This is a common problem in large-scale language model research, which manifests itself as the model producing unreasonably high confidence outputs when faced with certain situations. These outputs usually lack practical basis and mislead users.
This phenomenon is particularly prominent in natural language processing (NLP) tasks, such as automatic replies, translation, and text generation tasks. When AI models handle these tasks, they may produce absurd or meaningless answers, but these answers are still presented to users with high confidence. This not only affects the credibility of the model, but may also lead to serious misleading and error propagation.
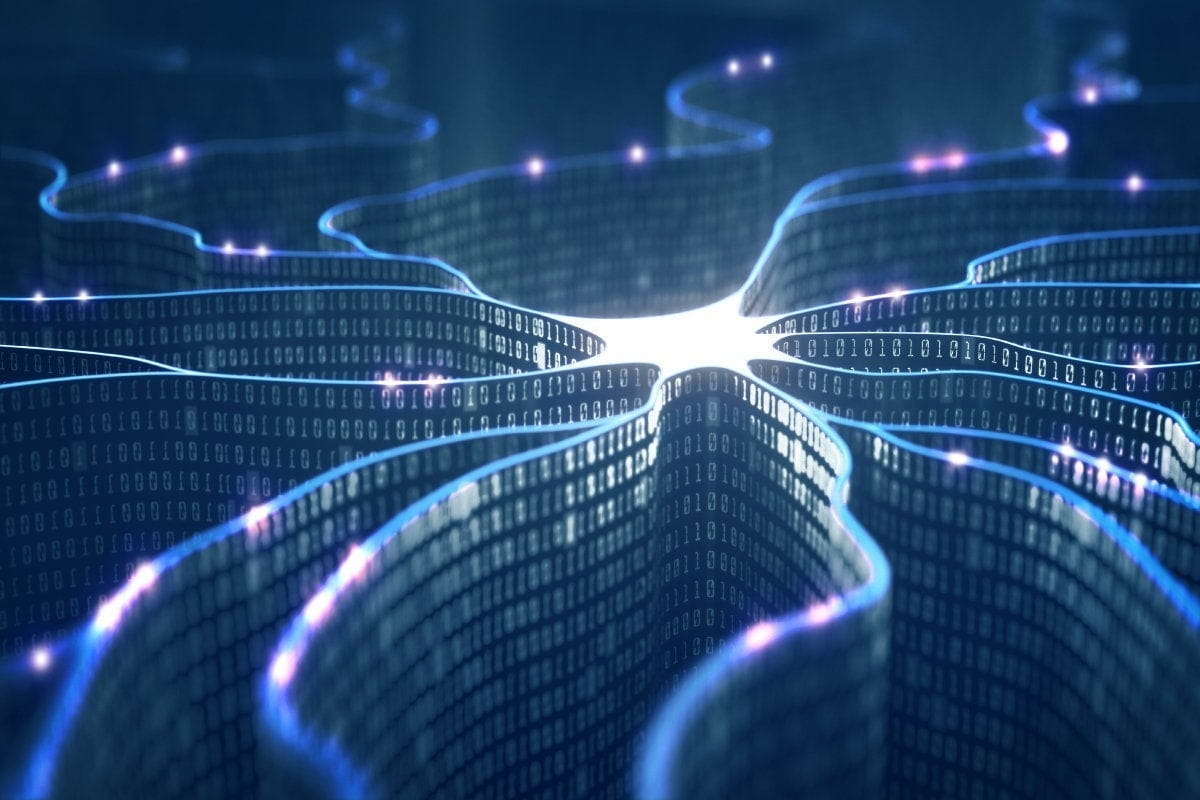
The Birth of the Woodpecker Tool
Determined to address this problem, a team of scientists from USTC and Tencent Youtu Lab developed the Woodpecker tool, whose name means correction and revision, to improve the quality and transparency of large multimodal language models (MLLMs).
The core idea of Woodpecker is to adopt a multi-model evaluation method, using three independent AI models, namely GPT-3.5 turbo, Grounding DINO and BLIP-2-FlanT5, as evaluators to identify hallucination phenomena and guide the models that need to be corrected to regenerate outputs. This multi-model evaluation method effectively increases cross-validation between models, thereby reducing the risk of misleading outputs.
How Woodpecker Works
The working principle of the Woodpecker tool can be divided into several key steps:
Data sampling and input: First, Woodpecker samples a set of data samples for evaluation, which contain various contexts and situations. These data samples are input into the AI models to be evaluated to obtain their outputs.
Multi-model evaluation: Next, Woodpecker uses three independent AI models, GPT-3.5 turbo, Grounding DINO, and BLIP-2-FlanT5, to evaluate these outputs. These three models are regarded as independent "referees" to detect whether there is a "hallucination" phenomenon.
Detecting hallucinations: If any of the three models believe that the model's output is hallucinating, Woodpecker will flag that output and determine that a correction is needed.
Regenerate output: For outputs marked as hallucinating, Woodpecker will guide the model to be evaluated to regenerate the output to ensure that the output is more reasonable, accurate and well-founded.
Transparency and improved accuracy: Finally, the introduction of Woodpecker provides additional transparency, ensuring that the model’s output is easier to understand. In addition, the researchers claim that the Woodpecker tool improves performance by 30.66% to 24.33% in terms of accuracy over the baseline model.
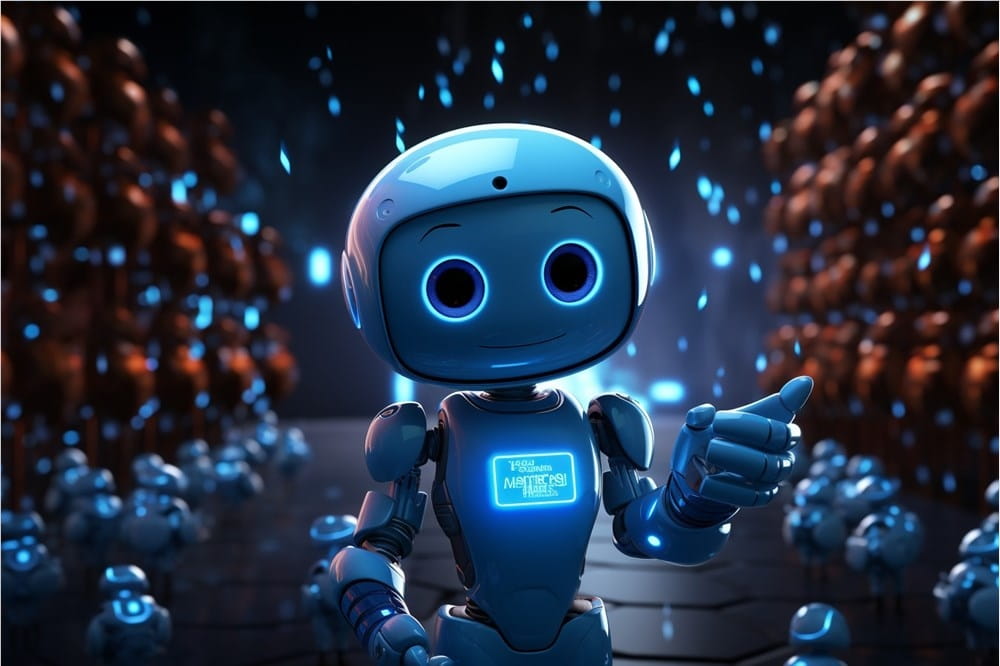
Application Areas
The application areas of Woodpecker tools are very wide, especially in tasks that require a high degree of accuracy and confidence. Here are some possible application areas:
Natural Language Processing: In areas such as automated responses, chatbots, and text generation, Woodpecker can help ensure that the model’s output is more reasonable and understandable.
Machine Translation: For machine translation tasks, Woodpecker can reduce misleading output in translation and improve translation quality.
Virtual Assistants: In virtual assistant applications, Woodpecker can help ensure that the answers provided by the assistant are accurate and not misleading the user.
Education: Woodpecker tools can be used in online education for automated answering systems to ensure students receive accurate feedback.
Medical diagnosis: In the medical field, Woodpecker can be used to assist doctors in making diagnoses and providing accurate medical advice.
Future Outlook
The Woodpecker tool represents a significant breakthrough in the phenomenon of "hallucination" in AI models. As AI technology continues to develop, we can foresee that this tool will be more widely used in the future. However, there are still some challenges to overcome. For example, the performance of Woodpecker may be limited by the evaluation model used, and the tool needs to be constantly updated to adapt to new data and contexts.
In addition, the development of Woodpecker also raises some ethical and privacy issues. For example, how does the tool handle users' personal information and data? How to ensure the protection of user data? These issues need to be properly addressed in the widespread application of the tool.
in conclusion
The birth of the Woodpecker tool marks the serious attention and resolution of the "hallucination" phenomenon in the AI field. This tool's multi-model evaluation method brings significant improvements to the transparency and accuracy of AI models, and is expected to help users better understand and trust AI systems in a variety of fields. However, as AI technology continues to evolve, we also need to continue to explore and solve related issues such as ethics and privacy to ensure the ethical use and sustainable development of AI technology. The Woodpecker tool is a big step forward in this effort, and we look forward to seeing it play an even greater role in future developments.