In their article “Financial Machine Learning,” Bryan T. Kelly and Dacheng Xiu explore the application of machine learning techniques in the study of financial markets. Published in July 2023, this article provides an overview of the emerging literature on the subject, highlighting the most promising examples and proposing future research directions. Intended for both financial economists wishing to understand machine learning tools and statisticians and machine learning specialists seeking interesting financial contexts to deploy advanced methods, this article is positioned as an essential reference in the field. The authors cite numerous previous works to support their analyses and recommendations, thus contributing to enriching the academic and practical debate on the integration of artificial intelligence in finance.
The aim of this article is to synthesize the main points covered in this paper, explaining how machine learning methods often outperform traditional econometric approaches and what challenges they pose to investors and financial analysts.
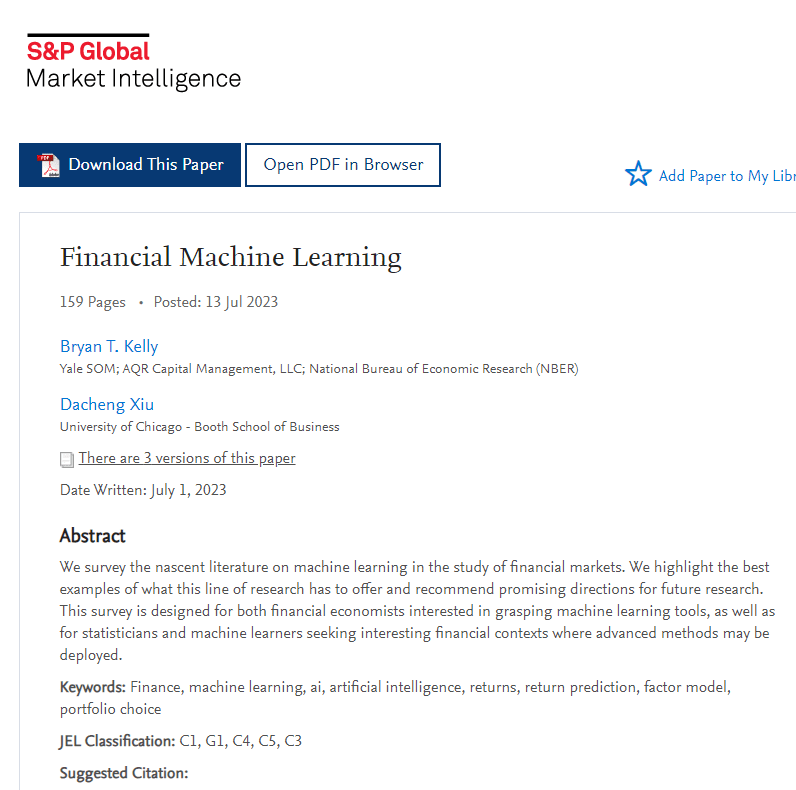
Application of Machine Learning Methods
One of the key aspects of this paper is the comparison between machine learning methods and traditional econometric approaches. The latter often rely on strong assumptions and simplified models to capture market dynamics. In contrast, machine learning techniques are designed to process massive data and detect complex patterns that traditional methods struggle to reveal.
Comparison with Traditional Econometric Methods
Traditional econometric models, such as linear regressions and ARIMA models, are widely used to predict financial returns and analyze relationships between variables. However, these methods have limitations, particularly in their ability to model nonlinearities and complex interactions. Machine learning, particularly neural networks and decision tree algorithms, is able to overcome these limitations by leveraging nonparametric and data-rich techniques to extract more detailed and predictive information.
Benefits and Challenges of Machine Learning
The advantages of machine learning lie in its ability to work with large amounts of data and learn adaptively. This allows for better detection of hidden patterns and anomalies. However, one of the main challenges is the risk of overfitting, where the model fits too well to historical data and loses generalization. In addition, these methods often require considerable computational resources and advanced technical expertise for their implementation.
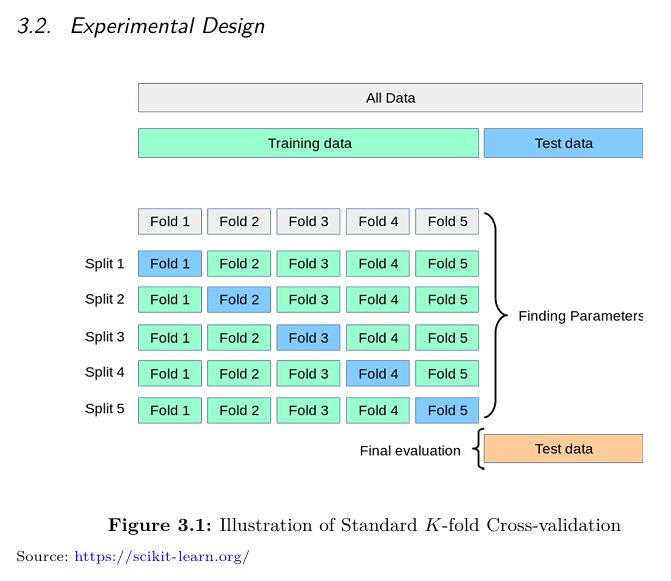
Complex and Parameter-Rich Models
Complex, parameter-rich models play a central role in machine learning analysis, particularly because they are able to capture non-linear relationships that simpler models miss. Financial markets are characterized by complex interactions between multiple variables, and this is where these models become important.
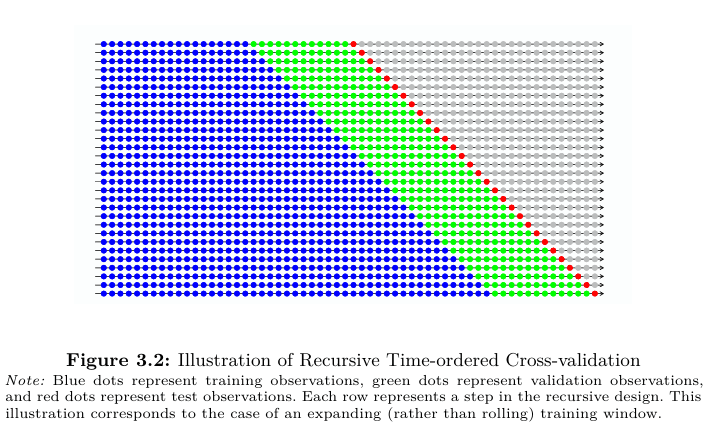
Improved Prediction Accuracy
Complex models, such as deep learning, use multiple layers of parameters to better fit data and produce more accurate predictions. This has immediate applications in price prediction, risk management, and portfolio optimization. In addition, they allow investors to better understand underlying market dynamics by identifying hidden trends.
Portfolio Optimization
With their ability to model complex relationships, these models also enable portfolio optimization by considering a broader range of factors. Traditional portfolio optimization often relies on models like Markowitz, but machine learning provides increased flexibility to adjust weightings based on changing market dynamics.
Financial Return Prediction Techniques
In the field of finance, some machine learning techniques stand out for their effectiveness in predicting financial returns. Among these, neural networks and decision trees are commonly used.
Neural Networks
Neural networks, inspired by the human brain, are capable of processing massive data sets and spotting complex patterns that are often inaccessible to traditional models. They are particularly used to predict future returns based on historical and real-time data, and their performance improves with the addition of new data.
Decision Trees and Forest Methods
Decision trees, and more specifically random forests, are powerful tools for classification and regression in financial markets. These models partition data into subsets based on criteria that maximize information, making them particularly effective at capturing nonlinear relationships between variables.
Risk-Return Balance
Another crucial aspect of using machine learning in finance is its ability to balance risk and return. Machine learning models can better assess risk by taking into account more complex and richer data than traditional models.
Risk Analysis and Portfolio Optimization
Machine learning enables portfolios to be adjusted based on market conditions in real time, optimizing the risk-return trade-off. This is particularly relevant in volatile environments where markets can change unexpectedly. Machine learning models enable faster responses and reduce potential losses while maximizing gains.
Practical Case Studies
In some studies, asset managers have used machine learning algorithms to manage their portfolios more efficiently, particularly in high-frequency trading strategies where responsiveness to market events is crucial.
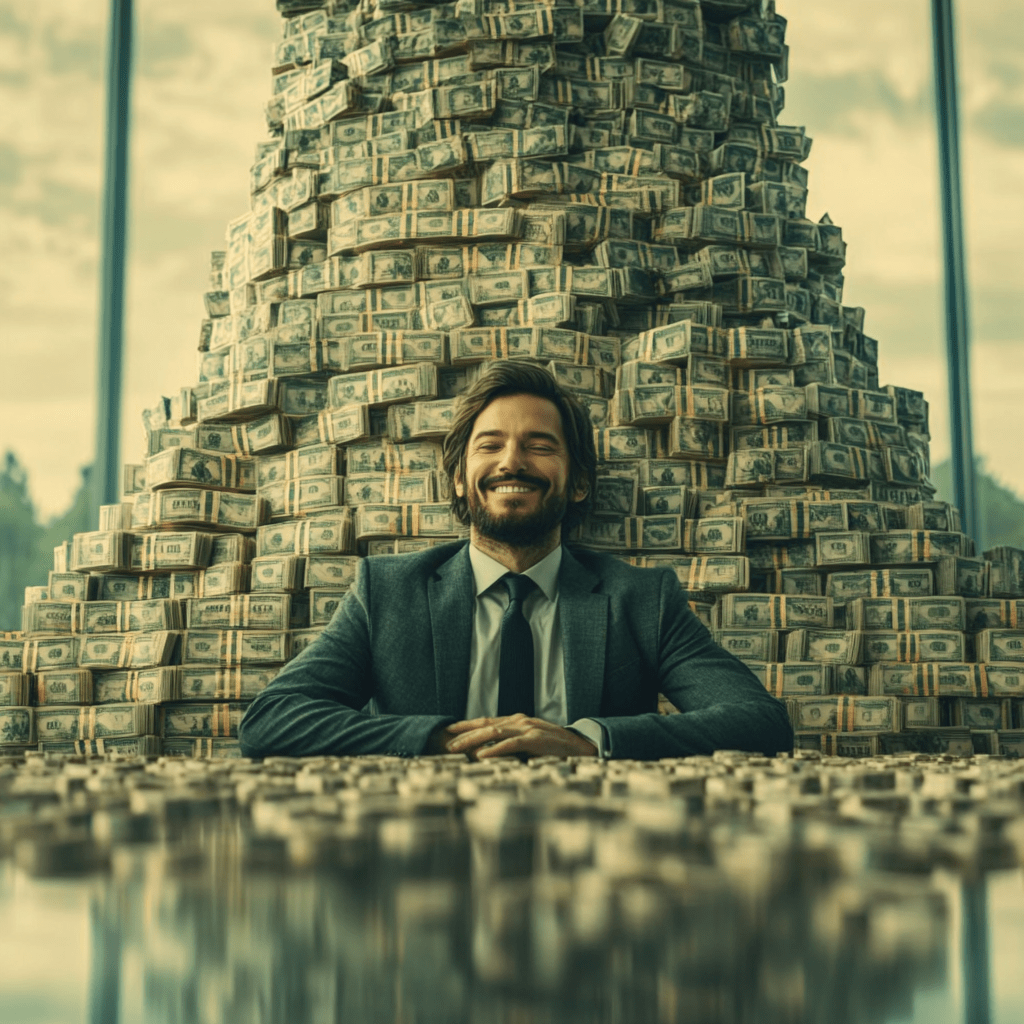
The Financial Machine Learning paper highlights the profound impact of machine learning on finance. These new methods offer unique perspectives to improve the accuracy of predictions, optimize portfolios and better manage risks. However, they also come with technical and operational challenges. For investors and financial analysts, the adoption of these tools is both an opportunity and a necessity in an increasingly data-driven sector. The future of finance clearly seems oriented towards the integration of these innovative technologies.
Disclaimer: This article does not constitute investment advice. Financial markets, and cryptoassets in particular, are volatile and involve risks. Always do your own research before making any financial decisions.
#IA #WeAreAllSatoshi #BTCUptober #FinancialUpdates #machinelearning