As OpenAI has launched AIGC models such as ChatGPT and Sora, it has led a new round of AI industry revolution. In the traditional computing power system, mainstream cloud computing service providers usually concentrate computing power relatively closed in multiple data centers consisting of hundreds of thousands of servers, so as to continuously provide computing services to the global network. Alphago, which once defeated Go master Lee Sedol, costs hundreds of thousands of dollars for a single training model. Companies like OpenAI that need to continuously train AIGC models have to pay computing costs that are astronomical figures that we ordinary people cannot imagine.
In the field of GPU computing power, Aethir @AethirCloud is one of the most representative DePIN projects in the field of GPU computing power. By building a DePIN system with GPU computing power as the core, it is committed to addressing the common challenges of centralized cloud computing, including high costs, GPU supply limitations, and latency. It also provides a decentralized GPU cloud service platform to provide long-term scalable solutions for rapidly growing markets such as AI, games, and rendering.
@AethirCloud network is one of the largest distributed GPU computing ecosystems currently. Computing resources will be accessed by enterprise users, Aethir partners and individual users in a distributed manner, which can efficiently meet the needs of the most difficult AI customers and provide enterprises with the highest quality GPU resources worldwide.
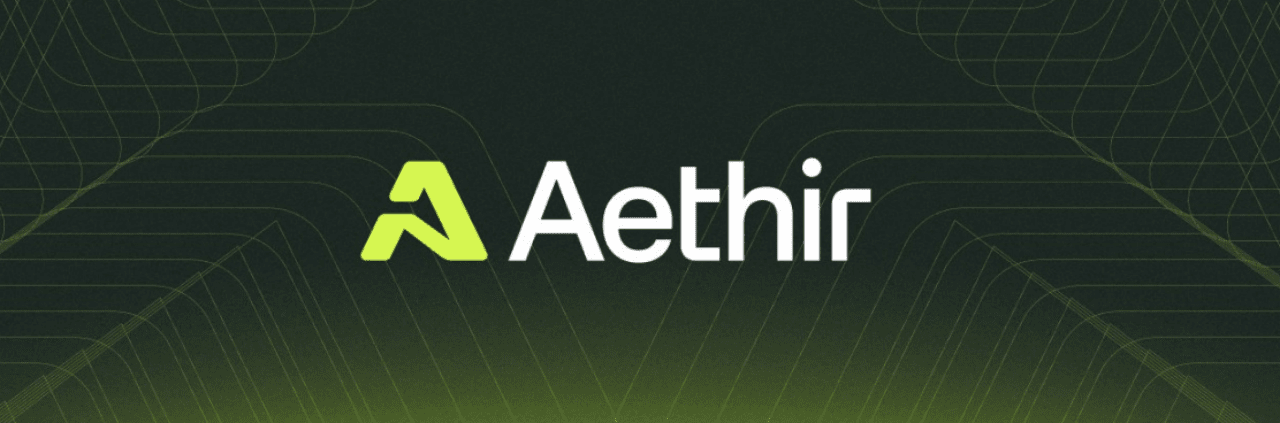
@AethirCloud itself is a DePIN network that aggregates GPU distributed computing resources and runs on Arbitrum. In the Aethir network, it allows users with computing resources to connect GPU computing power to the network and redistribute these computing powers in a distributed manner. Users with computing power needs can pay on demand through Wholesale, Retail, etc., while users who contribute GPU resources can earn income from the Aethir network.
On the supplier side, Aethir has a wide range. Telecommunications companies, hardware-intensive digital enterprise users, new infrastructure investors, and individual users with idle GPU computing resources can all access the network and contribute to it.
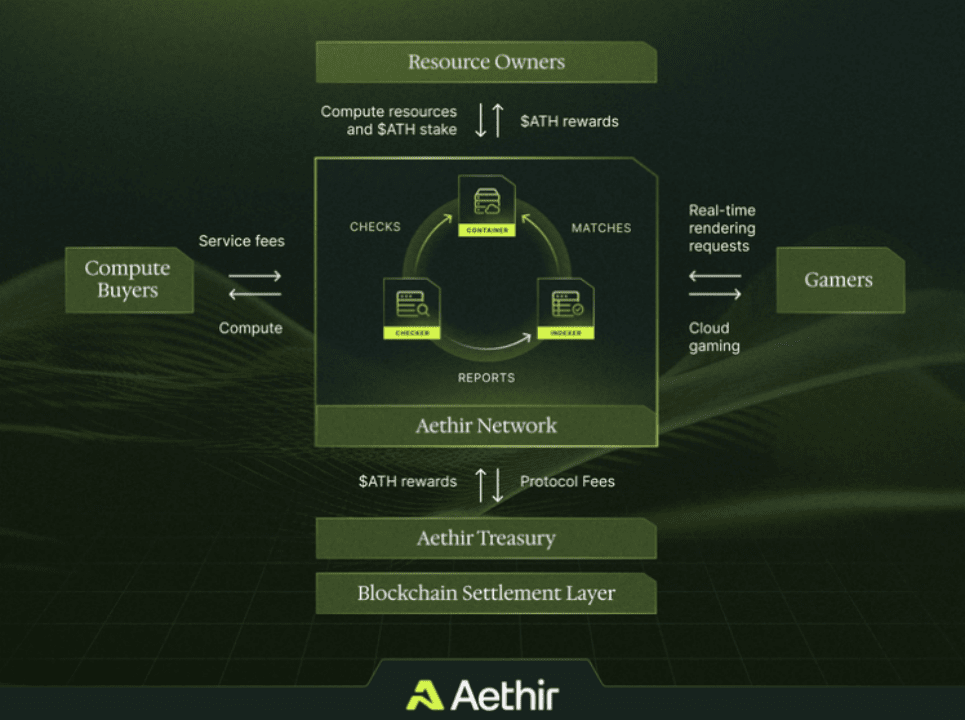
In fact, whether it is enterprises or individual users, GPU devices have more or less low utilization. At the same time, the ETH upgrade is very unfavorable to the development of PoW miners. After the merger is completed, a large number of PoW hardware devices are idle (the current scale calculation, the value of idle PoW computing resources is about 19 billion US dollars). From the supply and demand side, on the one hand, the global computing power is in short supply, and computing resource demanders cannot afford the expensive computing costs. On the other hand, GPU idleness leads to a large amount of computing power resources. Waste. Therefore, integrating idle GPU resources will be a huge computing resource pool, which is expected to alleviate the shortage problem faced by the computing field.
In fact, from the perspective of AI computing, there are also different sub-scenarios. These different scenarios have different requirements for computing power, which can be roughly divided into three categories:
One is AI training large models, which is often referred to as one of the most important forms of machine training. Large model training usually has extremely high requirements for computing power, and only NVIDIA is unique in this field.
One is AI reasoning, which is the process of using a trained AI model to make predictions or decisions. This process has relatively low requirements for computing resources.
Another type is some small edge models. This type of AI calculation usually does not require very high computing power.
Judging from the current GPU DePIN track landscape, due to limitations in GPU resources and scale, most projects in this track can only meet the second and third computing requirements mentioned above.
Aethir has a clear goal, which is to become the first DePIN project to launch Model as a Service, deploying machine learning models to the enterprise side for users to use. This allows AI users to complete the selection and rapid deployment of open source models in one stop. Aethir MaaS will help customers achieve efficient and intelligent data analysis and decision-making, and lower the threshold for model deployment.
In order to promote the development of the ecosystem in this direction, it is building a distributed computing cluster with NVIDIA H100 GPU as the core.
Relatively speaking, @ionet itself also has the ability to provide GPU computing power, but the quality and scale are far smaller than Aethir. Its target users are AI startups and developers, most of whom only need to perform reasoning or edge vertical model calculations rather than AI model training.
@akashnet_ is also a potential competitor in this direction, but Akash is good at CPU network clusters, which are more suitable for complex logic calculations, while GPUs are more advantageous in AI training, reasoning and other fields. Although Akash is also currently developing GPU computing clusters and has also introduced H100 (only about 140 cards), it still has a gap with Aethir in this direction.
In addition to the above projects, RNDR @rendernetwork, Gensyn @gensynai, etc. are also far behind Aethir in terms of GPU computing power, and it is difficult to form a direct competition with Aethir in the AI model training track. Therefore, scale is the advantage of Aethir MaaS system, and driven by its own structural network, it can be deeply integrated with more scenarios.
In addition to support for rendering and game latency, the excellent computing power of the Aethir GPU DePIN network is also expected to help online games build better security. Focusing on the field of online games, DDoS is the most common and frequent attack method, and all online games have paid a high cost to prevent DDoS. The Aethir GPU DePIN network can help online games resist real-time access attacks such as DDoS and ensure the continuous availability of game services.
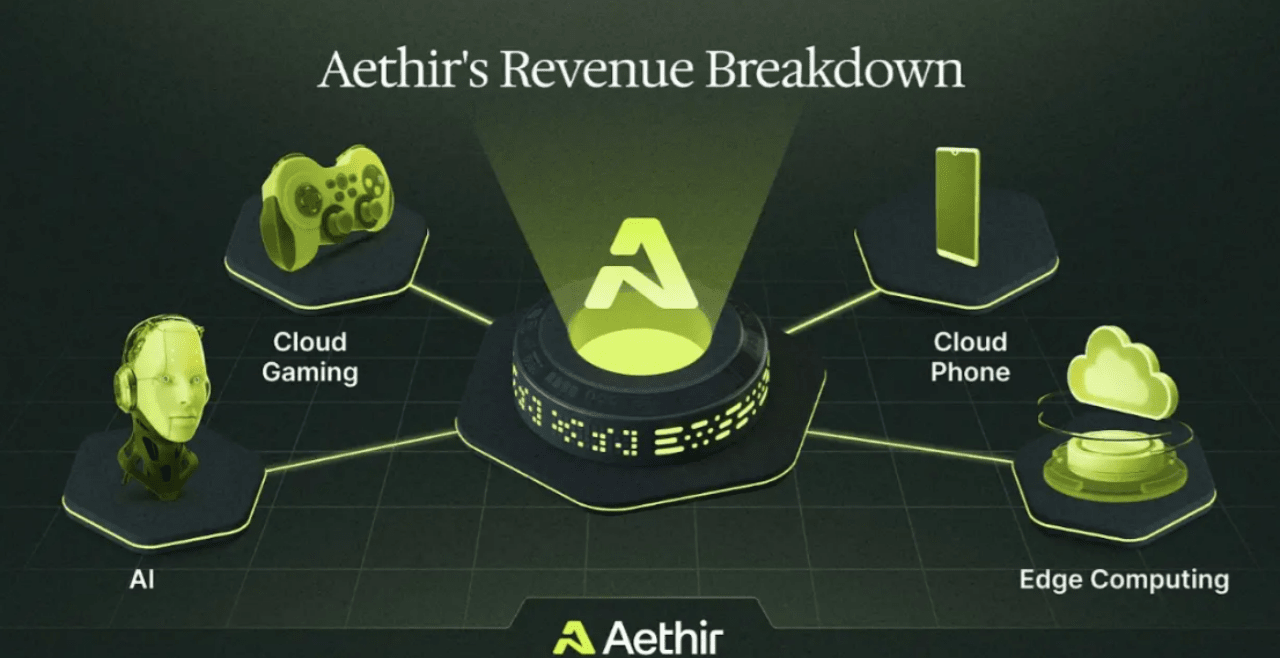
Thanks to its network architecture design, Aethir has certain advantages over most distributed GPU ecosystems in terms of latency, reliability, stability, and security. The Container role, which can flexibly drive computing power, has unlimited expansion capabilities, and is supervised in real time, allows Aethir to have unlimited expansion capabilities while being able to adapt to most scenarios with computing needs, rather than being targeted at a specific scenario.
For example, in addition to adapting to a series of scenarios such as AI, cloud rendering, and games, Aethir can also flexibly adapt to scenarios with extremely high requirements for latency, including autonomous driving, and some scenarios with extremely high requirements for computing. Therefore, the Aethir network itself can take root in the DePIN GPU computing track and continue to expand deeply into many scenarios with computing requirements.
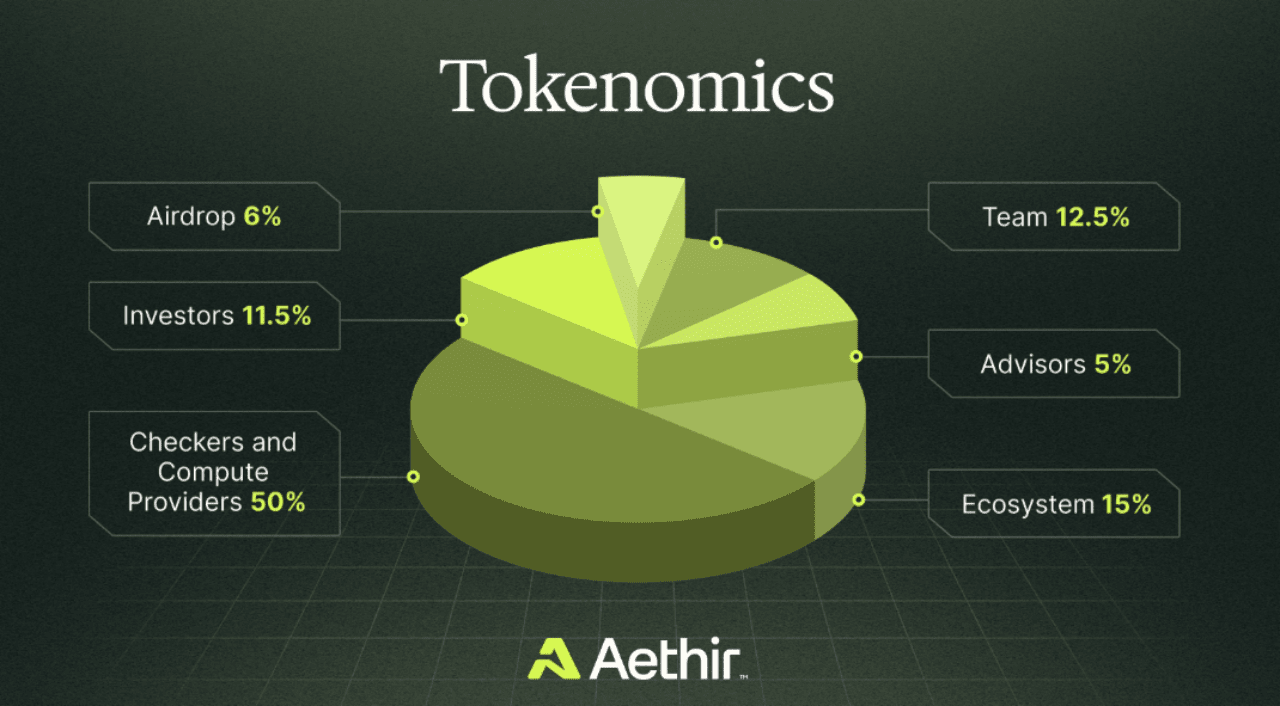
In fact, as the scale of the ecosystem grows, its decentralized ecosystem will continue to form a new growth flywheel. Similarly, according to a report by Precedence Research, with the increasing application of advanced technologies such as artificial intelligence and machine learning in cloud computing, the cloud computing market is expected to exceed the $1 trillion mark by 2028, which is a potential opportunity for the development of the Aethir ecosystem.